Since the rate of cyber threats seems rapid, conventional methods of cybersecurity have many times failed to meet them head on. Artificial intelligence and Machine Learning added a new perspective to the innovative technology of automation in cybersecurity to be able to handle new evolving kinds of threats. With this in mind, this paper examines how AI and ML transform cybersecurity practices, the part developers play in such transformation, and what should be considered best practices in the application of these technologies.
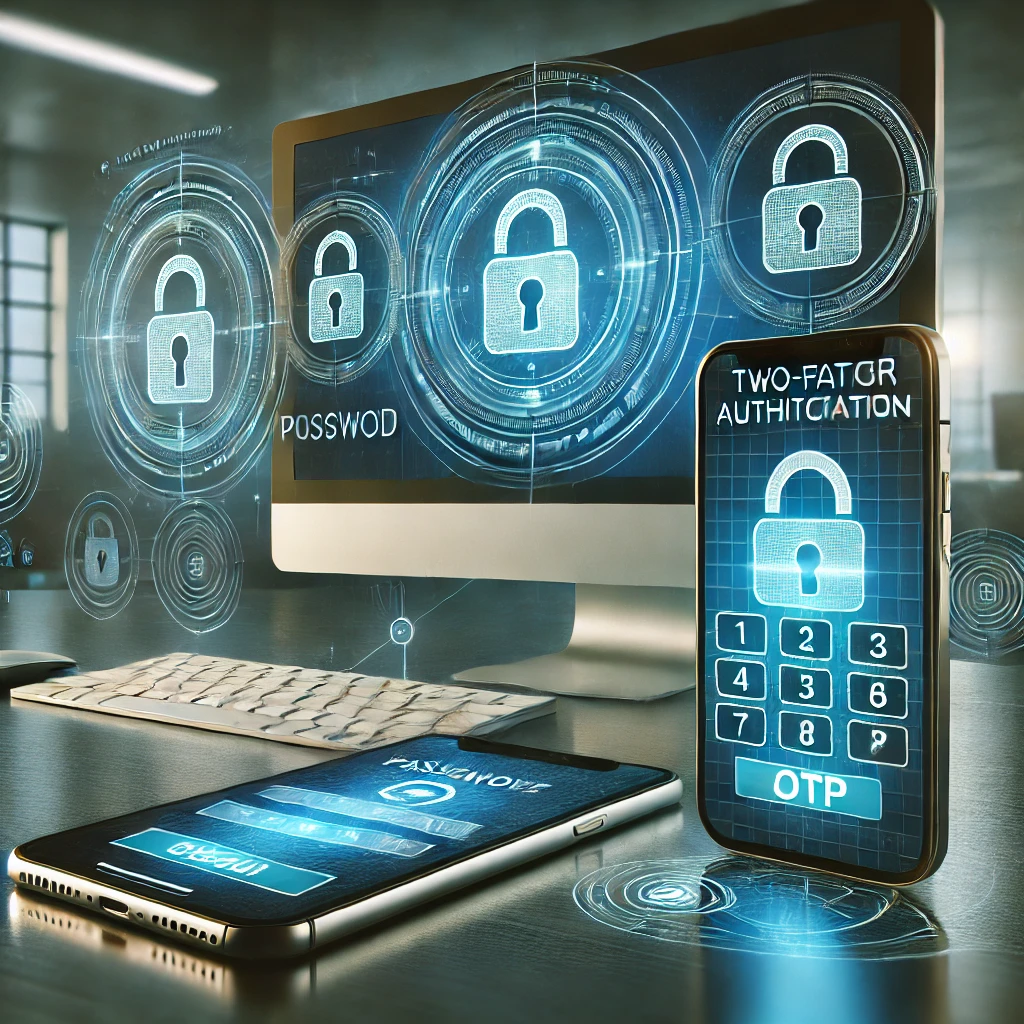
The Impact of AI and ML on Automation in Cybersecurity
AI and ML have structurally reinvented the way security practices operate by imposing automation and top-notch analytics. Machine Learning in cybersecurity authorizes massive data processing to identify patterns and anomalies that may show potential threats. Here’s how these technologies have been reinventing the field:
Traditional Threat Detection: Most forms of traditional threat detection rely on rules and signatures. AI-driven security automation tends to use ML algorithms while analyzing threats. These make the system learn from past data and make detections for new, previously unseen threats, turning the process of detection into a really dynamic one. This would permit automated threat detection that reduces response times while increasing accuracy.
AI for Security: More Than Threat Detection AI use cases go further than simple threat detection in cybersecurity. By applying AI, one can enhance security with the forecasting of potential vulnerabilities, correlation of risks associated with them, and providing automated incident response. It is highly important for constructing adaptive security systems featuring real-time responses against freshly emerging threats.
AI-Enabled Vulnerability Management: AI makes vulnerability management quite easier as it identifies and directly prioritizes the vulnerabilities based on the potential impact they may cause. In this way, security weaknesses could be managed in a much proactive and efficient manner.
Developer’s Role in AI-Enabled Security
A developer plays a critical role in integrating ML into cybersecurity practices. The role includes:
Integrating ML into Cybersecurity Practices: It demands the integration of machine learning models by developers within existing security infrastructures. This includes selecting appropriate ML algorithms for any type of threat analysis, training the model on relevant data, and finally integrating them into security systems.
Adaptive Security Systems: Designing of adaptive security systems will be left to the developers, where AI and ML technologies should be used to build the systems capable of constant evolution toward new threats. This requires extensive expertise in cybersecurity principles, as well as AI technologies.
AI in Security Protocols: It is about creating proper algorithms for correct identification and response in accordance with the organizational security policy to which the automated systems must adapt.
Best Practices in Cybersecurity Automation
Automated Cyber Defense Frameworks: Establish proper automated frameworks for cyber defense. This, of course, should stipulate the procedures for engagement with AI and ML technologies, and in the case of threat responses, such should be treated accordingly.
Cybersecurity Automation Tools: Avail yourself of cybersecurity automation tools, including AI and ML. These will facilitate nearly every aspect of cybersecurity, from the detection of threats through incident response and further into vulnerability management.
Developers’ Guide for ML-Based Incident Response: The developers should provide comprehensive guides to utilize ML-based incident response, including how to train and deploy ML models, best practices in handling the incidents detected by AI systems.
Challenges and Considerations
While there is a considerable benefit in leveraging AI and ML, there is also a consideration of challenges:
Biases in ML Models: The machine learning models might inadvertently learn biases within the training data, influencing its accuracy and effectiveness. Any model developed should be trained on diverse and representative datasets.
Integration Complexity: AI and ML integration to the existing security system may be complex and require considerable workflow and infrastructure changes.
Because of this, AI and ML are definitely not replacing human oversight. It is very important that the developers provide mechanisms for monitoring and validating AI-driven decisions with organizational security goals in mind.
Conclusion
AI and ML are revolutionizing cybersecurity automation. It’s finding better threat detection, vulnerability management, and automating incident responses. It is within these transformations that the developers develop by integrating ML models into security systems, building adaptive frameworks for cybersecurity. An organization following best practices and addressing challenges around AI and ML will also be able to begin exploring new ways of applying the technologies to construct even more robust and resilient security infrastructures. Since the cyber threat keeps growing continuously, embracing AI-driven automation would stand out as a major way of keeping pace with an ever-shifting landscape of cybersecurity.
Leave a Reply